View : 630 Download: 0
Machine learning-based prediction of response to growth hormone treatment in Turner syndrome: the LG Growth Study
- Title
- Machine learning-based prediction of response to growth hormone treatment in Turner syndrome: the LG Growth Study
- Authors
- Jung, Mo Kyung; Yu, Jeesuk; Lee, Ji-Eun; Kim, Se Young; Kim, Hae Soon; Yoo, Eun-Gyong
- Ewha Authors
- 김혜순
- SCOPUS Author ID
- 김혜순
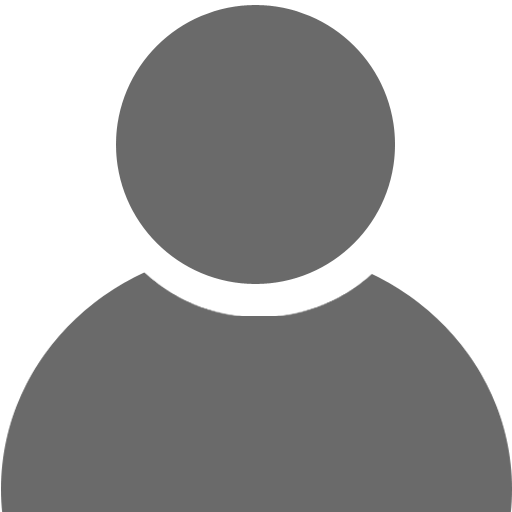
- Issue Date
- 2020
- Journal Title
- JOURNAL OF PEDIATRIC ENDOCRINOLOGY & METABOLISM
- ISSN
- 0334-018X
2191-0251
- Citation
- JOURNAL OF PEDIATRIC ENDOCRINOLOGY & METABOLISM vol. 33, no. 1, pp. 71 - 78
- Keywords
- growth hormone; height outcome; Turner syndrome
- Publisher
- WALTER DE GRUYTER GMBH
- Indexed
- SCIE; SCOPUS

- Document Type
- Article
- Abstract
- Background: Growth hormone (GH) treatment has become a common practice in Turner syndrome (TS). However, there are only a few studies on the response to Gil treatment in TS. The aim of this study is to predict the responsiveness to GH treatment and to suggest a prediction model of height outcome in TS. Methods: The clinical parameters of 105 TS patients registered in the LG Growth Study (LGS) were retrospectively reviewed. The prognostic factors for the good responders were identified, and the prediction of height response was investigated by the random forest (RF) method, and also, multiple regression models were applied. Results: In the RF method, the most important predictive variable for the increment of height standard deviation score (SDS) during the first year of GH treatment was chronologic age (CA) at start of Gil treatment. The RF method also showed that the increment of height SDS during the first year was the most important predictor in the increment of height SDS after 3 years of treatment. In a prediction model by multiple regression, younger CA was the significant predictor of height SDS gain during the first year (32.4% of the variability). After 3 years of treatment, mid-parental height (MPH) and the increment of height SDS during the first year were identified as significant predictors (76.6% of the variability). Conclusions: Both the machine learning approach and the multiple regression model revealed that younger CA at the start of GH treatment was the most important factor related to height response in patients with TS.
- DOI
- 10.1515/jpem-2019-0311
- Appears in Collections:
- 의과대학 > 의학과 > Journal papers
- Files in This Item:
There are no files associated with this item.
- Export
- RIS (EndNote)
- XLS (Excel)
- XML