View : 820 Download: 322
On-Demand Computation Offloading Architecture in Fog Networks
- Title
- On-Demand Computation Offloading Architecture in Fog Networks
- Authors
- Jin, Yeonjin; Lee, HyungJune
- Ewha Authors
- 이형준
- SCOPUS Author ID
- 이형준
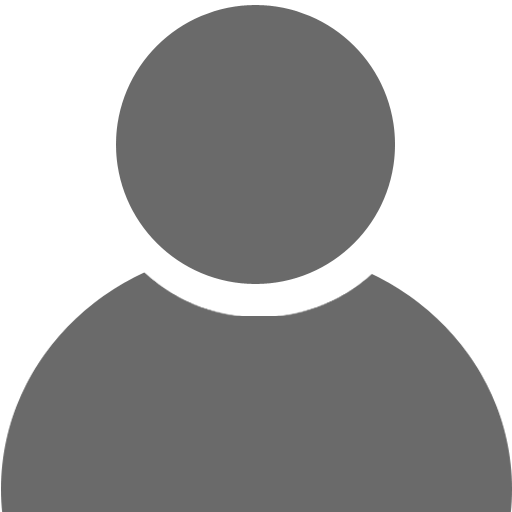
- Issue Date
- 2019
- Journal Title
- ELECTRONICS
- ISSN
- 2079-9292
- Citation
- ELECTRONICS vol. 8, no. 10
- Keywords
- computation offloading; in-network resource allocation; fog networks; edge computing
- Publisher
- MDPI
- Indexed
- SCIE; SCOPUS

- Document Type
- Article
- Abstract
- With the advent of the Internet-of-Things (IoT), end-devices have been served as sensors, gateways, or local storage equipment. Due to their scarce resource capability, cloud-based computing is currently a necessary companion. However, raw data collected at devices should be uploaded to a cloud server, taking a significantly large amount of network bandwidth. In this paper, we propose an on-demand computation offloading architecture in fog networks, by soliciting available resources from nearby edge devices and distributing a suitable amount of computation tasks to them. The proposed architecture aims to finish a necessary computation job within a distinct deadline with a reduced network overhead. Our work consists of three elements: (1) resource provider network formation by classifying nodes into stem or leaf depending on network stability, (2) task allocation based on each node's resource availability and soliciting status, and (3) task redistribution in preparation for possible network and computation losses. Simulation-driven validation in the iFogSim simulator demonstrates that our work achieves a high task completion rate within a designated deadline, while drastically reducing unnecessary network overhead, by selecting only some effective edge devices as computation delegates via locally networked computation.
- DOI
- 10.3390/electronics8101076
- Appears in Collections:
- 인공지능대학 > 컴퓨터공학과 > Journal papers
- Files in This Item:
-
On-Demand Computation Offloading Architecture.pdf(848.86 kB)
Download
- Export
- RIS (EndNote)
- XLS (Excel)
- XML